
This also has a lot for fine tuning retrieval for specific tasks by filtering the corpus to make it smaller. Thanks to this, researchers gain a lot more control than would be possible otherwise. That means other AI researchers can see into and control the corpus, so they can experiment with scaling and optimizing different methods to push retrieval technology forward
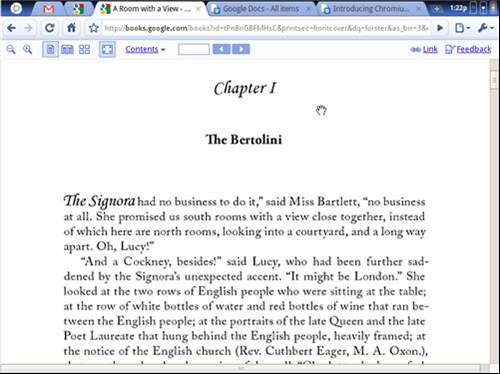
Our new knowledge source, Sphere, uses open web data rather than traditional, proprietary search engines.
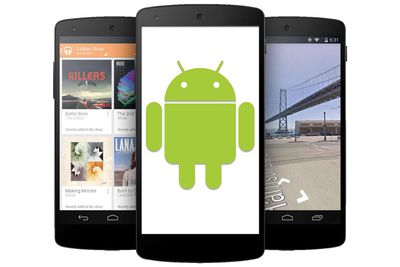
Instead they leverage the information available information online. They even refuse to black-box search engines in building their corpus. That is why they put a lot of effort into opening up everything. It also makes experimenting on these systems to test for security that much harder. Discussions around the topic can be limited since noone can go into the details. It can be hard for outsiders to scrutinize and test the solutions, which can lead to the propogation of biases. Why is that a problem? This turns these solutions into blackboxes of sorts. Meta becoming an unlikely champion of Open Machine Learning. This contributes to the AI replication crisis, which I covered here. There is no information given about how they were created, what data/preprocessing was applied etc.

Take Google’s Pathways architecture, that I broke down in May. Big Tech companies have been under a lot of scrutiny because of how opaque the ML research published by them is. One of the reasons that this development has a lot of Deep Learning insiders buzzing is the nature of it’s release. Believe it or not, 10 years down the line, this might end up being Meta’s biggest contribution to Deep Learning Research. There is one final thing that is very interesting about Sphere that has me very excited. Sphere seems to adapt to such tasks much better, most probably because of it’s larger+more chaotic corpus. In such a case, more traditional search archiectures don’t perform as well. Think of how often human search is vague/imprecise. This ability to not only parse through documents, but also make contextually aware inferences is why I think we might see a Sphere based search platform soon. Wikipedia based architecture is on the left and Sphere is on the right. How can the moderation team go through and check every article and make sure every citation is appropriate? Just from the start of 2022, 128,741 new English articles have been added to Wikipedia. However, it has grown to a scale where purely human moderation is impossible. The team at Wikipedia works hard to ensure that the quality of articles and citations is well maintained. One of the go-to sources of information is Wikipedia. A good creator has access to his audience's attention in a way that traditional media does not. This is one of the biggest reasons that creators have such a high-earning potential today. Even if I see a video with more views on the same topic, I am likely to with Wrath just because he has consistently put out the highest quality information when it comes to more advanced Math topics. Take the fantastic Math YouTube channel, Wrath of Math. Most people resort to relying on trusted sources of information. The amount of data created every day makes it impossible for any human vetting.
